FUN-PROSE: A Deep Learning Approach to Predict Condition-Specific Gene Expression in Fungi
Themes: Conversion
Keywords: Genomics, Modeling
Citation
Nambiar, A., Dubinkina, V., Liu, S., Maslov, S. Nov. 16, 2023. Data from: “FUN-PROSE: A Deep Learning Approach to Predict Condition-Specific Gene Expression in Fungi.” GitHub Repository.
Overview
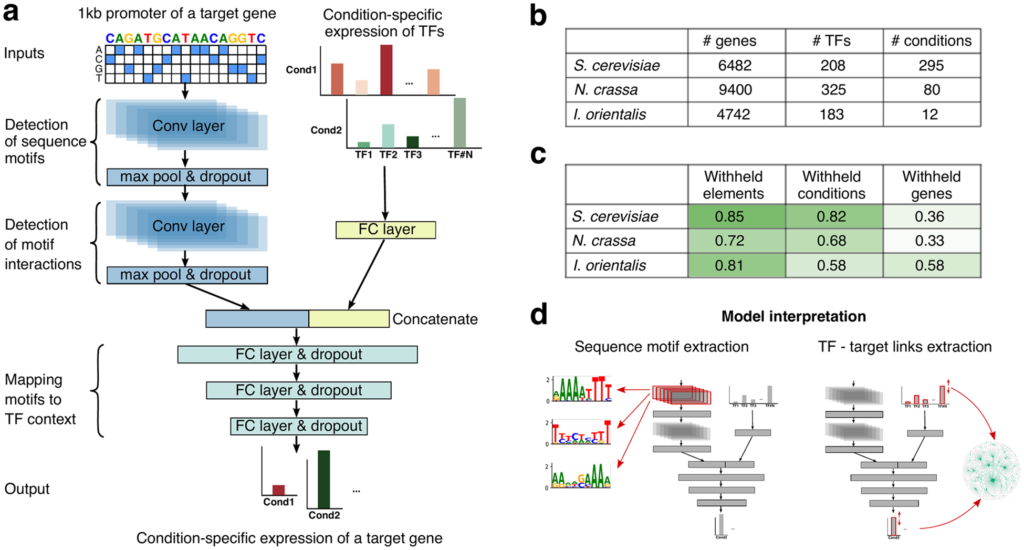
mRNA levels of all genes in a genome is a critical piece of information defining the overall state of the cell in a given environmental condition. Being able to reconstruct such condition-specific expression in fungal genomes is particularly important to metabolically engineer these organisms to produce desired chemicals in industrially scalable conditions. Most previous deep learning approaches focused on predicting the average expression levels of a gene based on its promoter sequence, ignoring its variation across different conditions. Here we present FUN-PROSE—a deep learning model trained to predict differential expression of individual genes across various conditions using their promoter sequences and expression levels of all transcription factors. We train and test our model on three fungal species and get the correlation between predicted and observed condition-specific gene expression as high as 0.85. We then interpret our model to extract promoter sequence motifs responsible for variable expression of individual genes. We also carried out input feature importance analysis to connect individual transcription factors to their gene targets. A sizeable fraction of both sequence motifs and TF-gene interactions learned by our model agree with previously known biological information, while the rest corresponds to either novel biological facts or indirect correlations.
Data
GitHub – FUN-PROSE: Includes code and data
Download (46.1KB) includes:
- Fungal dataset
- FUN-PROSE performance
- Cluster data