Deciphering Enhancer Sequence Using Thermodynamics-Based Models and Convolutional Neural Networks
Themes: Conversion
Keywords: Modeling, Transcriptomics
Citation
Dibaeinia, P., Sinha, S. Sept. 11, 2021. Data from: “Deciphering Enhancer Sequence Using Thermodynamics-Based Models and Convolutional Neural Networks.” GitHub Repository.
Overview
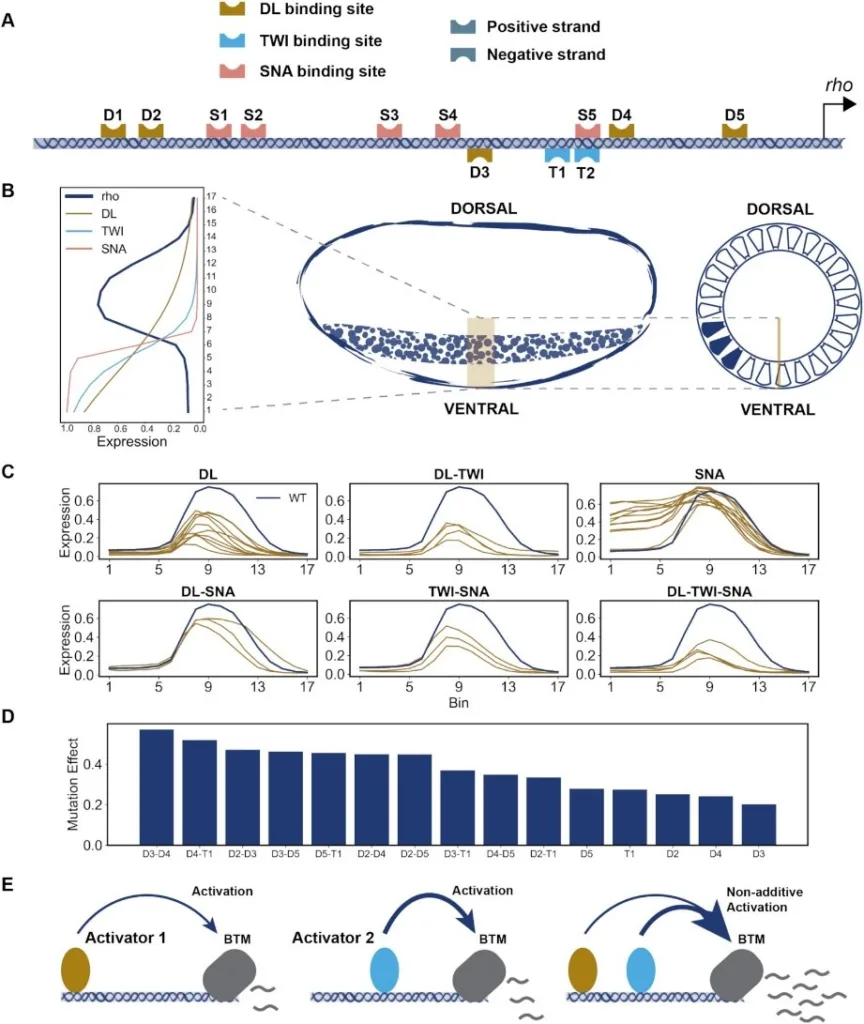
Deciphering the sequence-function relationship encoded in enhancers holds the key to interpreting non-coding variants and understanding mechanisms of transcriptomic variation. Several quantitative models exist for predicting enhancer function and underlying mechanisms; however, there has been no systematic comparison of these models characterizing their relative strengths and shortcomings. Here, we interrogated a rich data set of neuroectodermal enhancers in Drosophila, representing cis- and trans- sources of expression variation, with a suite of biophysical and machine learning models. We performed rigorous comparisons of thermodynamics-based models implementing different mechanisms of activation, repression and cooperativity. Moreover, we developed a convolutional neural network (CNN) model, called CoNSEPT, that learns enhancer ‘grammar’ in an unbiased manner. CoNSEPT is the first general-purpose CNN tool for predicting enhancer function in varying conditions, such as different cell types and experimental conditions, and we show that such complex models can suggest interpretable mechanisms. We found model-based evidence for mechanisms previously established for the studied system, including cooperative activation and short-range repression. The data also favored one hypothesized activation mechanism over another and suggested an intriguing role for a direct, distance-independent repression mechanism. Our modeling shows that while fundamentally different models can yield similar fits to data, they vary in their utility for mechanistic inference.